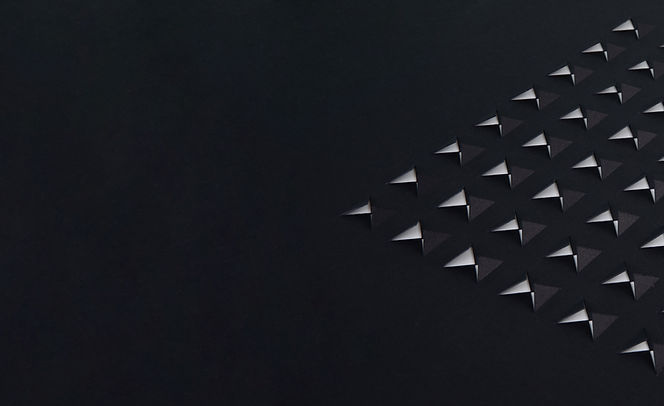
PROJECT HUB
WILD IDEAS AT FOUNDATIONS AND APPLICATIONS OF EMERGENCE (FAEME)
Here you can find a few inspirational projects that express the research interest of some of the researchers associated with FAEME. This project hub will be updated throughout 2022-2027.
Modelling learning and cooperation using evolutionary game theory
Understanding the emergence of learning and cooperation is a central question in human and animal group behaviour and has a strong impact in society and ecology. The microscopic description of such systems can be modelled using a chemical reaction network in which any two agents of the group interact (e.g. by exchange of information). By addressing what type of strategies can be chosen by the agents, it is possible to use a combination of stochastic methods and numerical simulation to understand what dominant strategies (cooperative or defective) emerge. We will test this against experimental data using moths in which the underlying network is a type of chemical communication network and information exchange is mediated via pheromones, as such uncovering the mechanisms by which moths learn. Relevant experimental data is also available in large scale gaming environments aimed at understanding economic/social behaviour.
Detecting emergence
Identifying and characterizing emergence is a non-trivial task in any given system. Using information theory, the renormalisation group and taking inspiration from parallel efforts in measuring complexity, this project will take pioneer steps to define measures of emergence and develop a model independent way of characterizing it.
Understanding the principles behind intelligent collective behaviour
Many systems share similar collective properties ranging from pattern formation in schools of fish to intelligent collective decision making in human societies. This project will use a combination of agent based modelling and Ginzburg-Landau theory to understand whether such collective behaviour can be characterized in terms of symmetry breaking patterns analogously to states of matter.
The basic principles of non-equilibrium driven, stochastic and active systems
There is currently no theory of far-from-equilibrium thermodynamics, except for some elements arising from stochastic thermodynamics and information theory. However, understanding these principles is at the heart of many problems including describing the microscopic behaviour of open systems such as many living and intelligent systems. This project will aim at uncovering these principles using a combination of multiscale modelling, hydrodynamic limits and large deviation theory.
Understanding the emergence of synergism and antagonism in evolutionary models
Red queen dynamics (host-symbiont interactions and evolution) is probably the main driving force in generating biodiversity on earth. These interactions can be synergistic, which can result in gene loss (reduction of redundancy in the system, as one of the interacting partners provides for both) and thus the evolution of interdependency (eukaryotic cells are a good example: merging of an Archaea with a bacteria, which have become completely interdependent). These interactions can also be antagonistic (host-pathogens; think of e.g. host-virus evolution), which can result in (accelerated) evolution of novel traits so as to escape attack. Main question: when do interactions become synergistic and/or antagonistic?
Optimal design of chemical communication using molecular simulations and machine learning
Chemical communication is the oldest form of communication in nature, yet the evolution of signals and responses is still poorly understood. Due to their lock-and-key mechanism and the fact that information transfer is only meaningful when specific, changes in the systems are suboptimal and generally selected against. Yet, e.g. olfactory receptors are among the fastest evolving genes in the animal kingdom. Through molecular simulations and machine learning, we aim to get a better understanding of possible evolutionary pathways.
Is there space and time for experimental philosophy?
This project will work on three questions. Can modern approaches describing the physics of emergent electronic quantum phases such as AdS/CFT interface and interact with philosophical research into emergence? Can this incubate a new field of ‘experimental philosophy’? What can the experimental exploration of emergent quantum phases and its philosophical underpinning teach us about emergence in general? This nascent connection between experimentally measured behaviour of electrons in the lab and questions of current interest to philosophers has got a huge boost due to the relevance of theoretical tools provided by the AdS/CFT correspondence. Very recently, experimental data from the Amsterdam labs on so-called strange metals found in high temperature superconductors have been discovered to be better described by AdS/CFT routes than by any conventional condensed matter theory. This project will work on connecting laboratory physics on emergent systems and philosophy of emergence. The aim is to move towards a new ‘experimental philosophy’ paradigm.
Bridging the gap between microscopic and macroscopic evolution
Translating microscopic processes to macroscopic patterns is one of the major challenges in many emergent systems, including in evolution. Can we find common features in chemistry, material science or other fields combinable with evolutionary biology so as to build common/overlapping hypotheses?
Information filtering in relation to development and environmental cues
The type of information that is perceived depends on previous experience and knowledge. This is exemplified currently by humans using different social media channels, but most likely also true in many systems/organisms in nature. This project explores whether patterns in human social-media-use can give insights into similar processes in nature.
Spectroscopic AI as an emergent topological metal detector
World-wide, photon science facilities generate huge quantities of data on (quantum) materials. Using a combination of AI-based approaches, the experimental spectroscopic data and materials theory, a powerful machinery for the discovery of new topological and other emergent quantum materials will be developed.
Emergence of causality via information flow in metastable transitions
Complex systems, from atomistic to planetary scales, often exhibit tipping points between metastable states. Understanding how these transitions can be endogenously generated (e.g. through induction by noise) is important for enabling control by intervention. However, as complex systems consist of many degrees of freedom it is often not evident which features are involved, and at which time, in the causation leading to a transition. New methodology based on information flows in dynamical networks can shed light on these issues. In this project we will apply such methodology on complex molecular transitions such as protein folding to find out how causality in such systems emerges from the microscopic degrees of freedom.
About the learnability of emergent features of chaos
It is an open question what type of features of a system can be effectively learned with techniques like machine learning (ML) type techniques, and which ones cannot. As a first attempt to develop some intuition for this question, we will study the mixed-field Ising model which is known to possess integrable and chaotic regimes in its parameter space. Both the energy spectrum and simple correlation functions will be used as data to investigate which features of the theory can be uncovered using ML techniques and which ones cannot.
A simple geobiochemical network to model Earth as a living system
Simple building blocks will be used to model the Earth as a living system using elements from geophysics (interior/surface/atmosphere), including cycles and (energy) balances. Various tests will be performed including how the system self-regulates and leads to potential instabilities, tipping points, and responds to major changes in the environment. This will provide a toy model of the environment that can help understand the emergence of life and evolution.
Understanding the emerging 4D structure of atmospheres from an inverse problems perspective
This project pits itself against the multi-scale inverse problem (spatial and temporal), and uses observations to retrieve parameters of the micro-macro descriptions of the atmosphere. It includes the application of statistical physics, as well as data assimilation methods. Uncertainties will be estimated using advanced uncertainty quantification methods.
Holistic view on group dynamics: feedback loops between system and subsystem/observer and effector
A number of similar emergent features of group dynamics can be encountered in many different fields of scientific inquiry. In this abstract, we only mention two of them. (1) One of these features is the way in which a supersystem may encode information that is not reducible to the sum of the information carried by its component subsystems. This is due to the existence of higher-level correlations. Examples are in physics (entanglement in quantum mechanics), biology, ecology and environmental studies (e.g. complex feedback loops that form an ecosystem), and social sciences (e.g. complex social behaviour, such as the so-called `wisdom of the crowds' or its opposite: informational cascades, pluralistic ignorance etc). (2) Another such feature is the way an observing system interferes and affects the behaviour of the observed system. Again, examples can be found in physics (the disturbance caused by quantum measurements), biology and ecology (where our probes and observations can easily contaminate an environment, or otherwise affect the behaviour of the observed species, even to the point of extinction) and social-cultural studies (the so-called ``Observer Effect"). The mathematical formalism developed in physics to study these phenomena is based on Hilbert spaces, with the first feature (1) above being captured by the use of tensor product instead of Cartesian product, and the second feature (2) above being formalized in terms of the non-commutativity of certain operators. In recent years, this formalism has been generalized in different directions: algebraic (using lattice-theoretic or category-theoretic structures) and logical/model-theoretical (using abstract transition systems as models for dynamics, and relational-epistemic structures as models for ``knowledge" in the sense of qualitative-semantic information). Such models, having a high level of generality, hold the promise for providing a unified approach to the above-mentioned emergent features of group dynamics, an approach that goes beyond the specific details of each of the above fields, in order to capture the common ``logic of group dynamics" that underlies these surface similarities. This project aims to take an overview of this line of research, illustrate it with examples from various areas of science, and articulate the potential benefits and goalposts, as well the main challenges, of this unified abstract approach.
Emergence and deep learning
Neural networks learn representations, mappings from input data to features. One of the central questions in AI research is how we can learn mappings from low-level features to high-level (abstract) features. Some forms of abstractions already emerge automatically through the process of learning, both in supervised (for a specific prediction task) as well as unsupervised learning (density estimation). For example, it is well known that the deeper layers in a convolutional neural network encode more abstract concepts than the lower layers. Just like is observed in the mammalian cortex, lower layers encode edges and corners in image data, while deeper layers encode for entire objects. A big open question on the road to “general” artificial intelligence is how more higher-level conceptual reasoning can emerge from neural representations (e.g. how do we represent the concept of “loading a dishwasher” or a “party game”, which can have varying concrete instantiations that are strongly dependent on context). One current line of thinking is that this will require models that perform “predictive processing” to successively reason about feature representations at higher and lower levels of abstraction, which in a sense is a hierarchical approach to emergence.